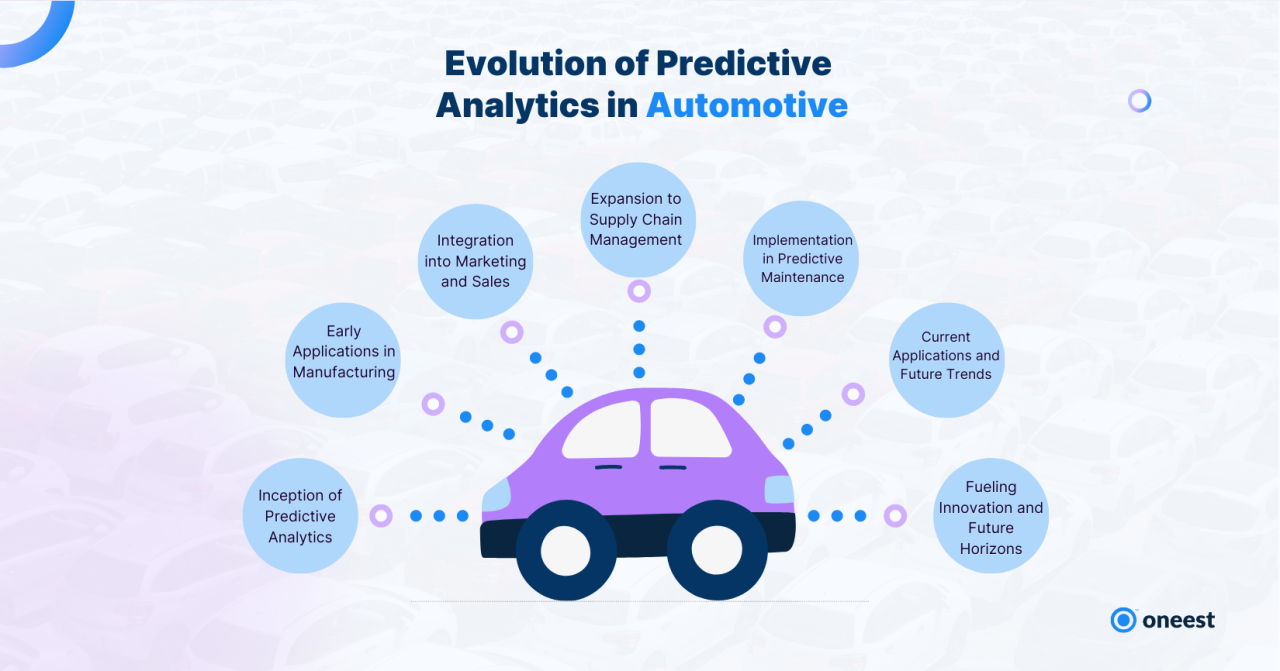
Automotive Predictive Analytics
Automotive Predictive Analytics is a game-changer in the automotive industry, offering insights into maintenance, performance optimization, and cost reduction. Dive into the world of predictive analytics in vehicles and discover the power it holds.
Overview of Automotive Predictive Analytics
Automotive predictive analytics is a method that uses data, statistical algorithms, and machine learning techniques to predict future outcomes in the automotive industry. It plays a crucial role in improving vehicle maintenance, performance optimization, and overall efficiency in the sector.
Utilization in Vehicle Maintenance and Performance Optimization
Predictive analytics in automotive helps in predicting potential issues with vehicles before they occur, allowing for proactive maintenance. By analyzing historical data on vehicle performance, manufacturers and service centers can anticipate when components may fail and schedule maintenance accordingly. This not only reduces downtime but also improves safety on the road.
Benefits of Implementing Predictive Analytics in the Automotive Sector
- Enhanced Vehicle Reliability: By predicting maintenance needs, vehicles can be kept in optimal condition, reducing breakdowns and unexpected repairs.
- Cost Savings: Proactive maintenance based on predictive analytics can lower repair costs and prevent major failures that may result in expensive repairs.
- Improved Safety: Predictive analytics can help identify potential safety hazards before they escalate, ensuring the safety of drivers, passengers, and pedestrians.
- Optimized Performance: By analyzing data on vehicle performance, manufacturers can fine-tune designs for better efficiency and performance.
Data Collection and Processing
In automotive predictive analytics, data collection and processing play a crucial role in deriving valuable insights and making informed decisions. This process involves gathering data from various sources, cleaning and preparing it for analysis, and addressing the challenges associated with handling large volumes of data.
Types of Data Sources
- Telematics systems: Utilizing data from sensors embedded in vehicles to track performance metrics such as speed, mileage, and fuel efficiency.
- Connected vehicles: Gathering data from vehicles equipped with internet connectivity to monitor real-time information like GPS location and engine diagnostics.
- Manufacturing data: Analyzing data from the production line to improve quality control and predict maintenance issues in vehicles.
Collecting and Preprocessing Data
- Data Collection: Automotive companies collect data from various sources such as onboard sensors, GPS devices, and customer feedback to create a comprehensive dataset.
- Data Cleaning: The collected data undergoes cleaning processes to remove duplicates, correct errors, and ensure consistency for accurate analysis.
- Data Integration: Combining data from different sources to create a unified dataset that can be used for modeling and predictive analytics.
Challenges in Data Collection and Processing
- Volume of Data: Dealing with large amounts of data generated by connected vehicles and manufacturing processes can be overwhelming and require advanced processing techniques.
- Data Quality: Ensuring data accuracy, completeness, and relevance is crucial for obtaining reliable insights and making accurate predictions.
- Data Privacy and Security: Protecting sensitive information collected from vehicles and customers to maintain trust and compliance with data regulations.
Machine Learning Models in Automotive Predictive Analytics
Machine learning plays a crucial role in automotive predictive analytics by enabling the development of models that can predict maintenance needs and potential failures in vehicles.
Common Machine Learning Algorithms in Automotive Predictive Analytics
Various machine learning algorithms are utilized in automotive predictive analytics to analyze data and make accurate predictions. Some common algorithms include:
- Linear Regression
- Random Forest
- Support Vector Machines (SVM)
- Gradient Boosting
- Neural Networks
Training and Deployment of Machine Learning Models for Predictive Maintenance
Machine learning models in automotive predictive analytics are trained using historical data on vehicle performance, maintenance records, and sensor readings. These models are then deployed to predict when components may fail or require maintenance based on real-time data.
Role of Real-Time Data Processing in Improving Predictive Analytics Models
Real-time data processing is essential in improving the accuracy of predictive analytics models in the automotive industry. By continuously analyzing data from sensors and monitoring vehicle performance in real time, machine learning models can make more precise predictions and help prevent unexpected breakdowns or failures.
Predictive Maintenance in Automotive Industry: Automotive Predictive Analytics
Predictive maintenance is a proactive approach that uses data analytics to predict when maintenance should be performed on a vehicle before a breakdown occurs. This concept is crucial in the automotive sector as it helps prevent unexpected failures, reduces downtime, and ultimately saves costs for companies.
Applications of Predictive Maintenance in Vehicles, Automotive Predictive Analytics
- Monitoring engine performance: By analyzing data from sensors, automotive companies can predict when certain engine components may need maintenance or replacement.
- Tire health monitoring: Predictive analytics can be used to monitor tire wear and predict when tires need to be replaced, preventing blowouts and accidents.
- Brake system analysis: By analyzing braking patterns and performance data, predictive maintenance can anticipate when brake pads or rotors need to be serviced, ensuring optimal safety.
Benefits of Predictive Maintenance for Automotive Companies
- Reduced downtime: By addressing maintenance issues before they lead to breakdowns, predictive maintenance helps keep vehicles on the road and minimizes unscheduled downtime.
- Cost savings: Predictive maintenance allows companies to plan and budget for maintenance tasks in advance, avoiding costly emergency repairs and replacements.
- Improved safety: By proactively addressing maintenance needs, predictive maintenance enhances vehicle safety and reduces the risk of accidents due to mechanical failures.
Top FAQs
How does predictive analytics benefit the automotive industry?
Predictive analytics helps in predicting maintenance needs, optimizing vehicle performance, and reducing downtime and costs for automotive companies.
What are the common challenges in data collection for automotive predictive analytics?
Challenges include dealing with large volumes of data, ensuring data quality, and integrating data from various sources.